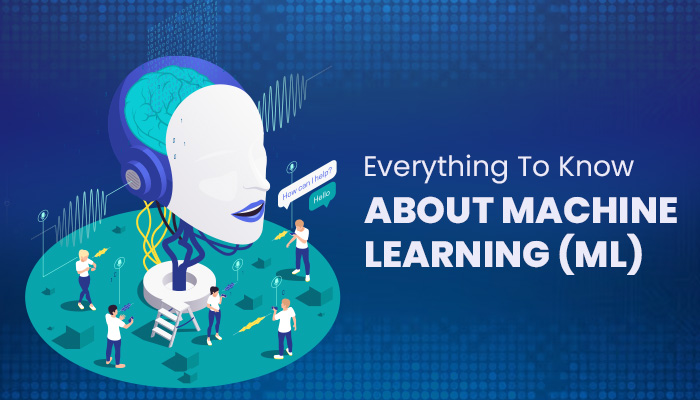
Everything To Know About Machine Learning
Machine Learning (ML) is a game-changing innovation to revolutionize various industries and businesses across the global market. It is a part or subset of artificial intelligence. ML trains machines to learn from experience for improving performance without explicit programming. This blog on Machine learning guide readers to information about intricacies, fundamental concepts, applications, and real-world impact.
We will render a comprehensive overview through this Machine learning guide about its core principles and how it differs from traditional programming. This blog will also focus on the types of ML and uses of machine learning across industries. From healthcare and finance to marketing and autonomous vehicles. We also rendered details related to the ethical considerations surrounding ML adoption.
As ML becomes increasingly integrated into the daily lives of users, it is also essential to address concerns regarding bias, transparency, and data privacy. Understanding and learning about these ethical concerns is critical for developing an accountable ML solution to ensure accountability and safety.
What is Machine Learning (ML)?
It is referred to as a subset of AI to enable computers and systems to learn and improve from experience without being explicitly programmed. Instead of following strict pre-defined instructions, ML algorithms enable machines to analyze data, identify patterns, and make predictions or decisions based on the insights gained.
The core principle of ML lies in its ability to learn and adapt autonomously, continuously refining its performance as it encounters new data.
In simple terms, machine learning allows computers to identify hidden patterns and relationships in vast datasets to recognize objects, understand language, predict outcomes, and even make complex decisions.
This technology has transformative potential across industries, as it enables businesses and researchers to harness the power of data-driven insights and automation for enhanced efficiency and innovation.
Types of Machine Learning
The machine learning guide here will also shed light on the types of ML present in the market. They are categorized into three main types based on the learning approach and the types of data used for training. We have mentioned them below:
-
Supervised Learning
This algorithm is trained on labeled data, where the input data and corresponding output labels are rendered. The algorithm learns to map input to output by uncovering patterns in the data. It is utilized for tasks like classification and regression, where the goal is to predict the correct label or value for new input data.
-
Unsupervised Learning
This one works with unlabeled data i.e., there are no explicit output labels provided during training. The algorithm learns to identify patterns and structures within the data without guidance. It is utilized in clustering, dimensionality reduction, and anomaly detection.
-
Reinforcement Learning
In reinforcement learning, the algorithm learns through trial and error by interacting with an environment. It receives feedback in the form of rewards or penalties based on its actions and learns to take actions that maximize the cumulative reward over time. It is utilized in conditions with no pre-defined training data to learn and make decisions in a dynamic and changing environment.
Uses of Machine Learning/Application of Machine Learning
Machine Learning is used and applied in various industries and fields. Some of the uses and applications of Machine Learning include:
-
Image & Speech Recognition
It is used in developing systems for accurate recognition and interpretation of images, videos, and speech. It is applied in facial recognition, object detection, speech-to-text, and voice assistants.
-
Natural Language Processing (NLP)
This enables machines to understand, interpret, and generate human language and is usually used in chatbots, virtual assistants, language translation, sentiment analysis, and text summarization.
-
Recommendation Systems
Machine Learning powers recommendation engines used in e-commerce, streaming platforms, and social media to analyze user behavior and provide personalized suggestions for improvement in user engagement.
-
Fraud Detection
Machine Learning algorithms can identify patterns and anomalies in financial transactions, enabling fraud detection and prevention in banking and online payment systems.
-
Healthcare
Machine Learning is used in medical image analysis, disease diagnosis, drug discovery, personalized treatment plans, and patient monitoring, improving healthcare outcomes.
-
Autonomous Vehicles
Machine Learning plays a crucial role in self-driving cars and autonomous vehicles, enabling them to perceive their surroundings, make decisions, and navigate without human intervention.
-
Financial Modeling
Utilized for credit risk assessment, stock market prediction, algorithmic trading, and customer service in the financial industry.
-
Predictive Maintenance
Utilization in predictive maintenance to detect equipment failures and schedule maintenance before breakdowns occur, reducing downtime and operational costs.
-
Personalization
Machine Learning is applied in content recommendation, marketing, and user experience personalization, enhancing customer satisfaction and retention.
-
Social Media Analysis
The algorithms analyze social media data to extract in-depth insights, and sentiment analysis, and identify trending topics for marketing and public opinion monitoring.
Ethical Issues Related To Machine Learning
Machine Learning (ML) comes with its own set of ethical issues that need to be carefully addressed to ensure the responsible and fair use of this technology.
Some of the key ethical concerns surrounding ML include:
-
Bias & Fairness
ML algorithms are trained on historical data, which can sometimes contain biased information. If not properly managed, ML models can perpetuate existing biases, leading to unfair treatment of certain groups or individuals.
-
Privacy & Data Protection
ML systems often require large amounts of data to function effectively. This raises concerns about the privacy and security of personal information used in these systems, necessitating proper data anonymization and consent mechanisms.
-
Accountability & Transparency
ML models can be complex and difficult to interpret. This lack of transparency can raise concerns about how decisions are made and can be challenging to hold ML systems accountable for their actions.
-
Autonomy & Control
As ML systems become more sophisticated, there is a risk of them acting autonomously without adequate human oversight. The potential loss of control over ML systems raises questions about responsibility and liability.
-
Job Displacement
ML's automation capabilities hold the potential to displace human workers from certain roles, leading to job losses and economic disruption. Ensuring a fair transition and retraining opportunities is crucial.
-
Manipulation & Deepfakes
ML can be used to create realistic fake videos, images, or audio, known as deepfakes. This raises concerns about the spread of misinformation, propaganda, and potential harm to individuals and society.
-
Autonomous Weapons
The use of ML in military applications and autonomous weapons raises ethical questions about the potential for machines to make life-and-death decisions without human intervention.
-
Consent & Data Ownership
ML often requires large datasets for training. The ownership of data and obtaining informed consent from individuals whose data is used is a critical ethical consideration.
-
Long-Term Impact
The rapid advancement and widespread deployment of ML raise questions about its long-term impact on society, the economy, and the environment.
Addressing these ethical issues requires collaboration between technologists, policymakers, ethicists, and society at large. Implementing guidelines and best practices for ethical ML development and usage can help ensure that this powerful technology is used responsibly and for the greater good.
Conclusion
The above discussion on Machine Learning guide helps the reader for a deep understanding of this transformative technology. ML, a subset of artificial intelligence, empowers machines to learn from data and make intelligent decisions, revolutionizing industries and businesses worldwide.
Understanding the types of ML in the Machine learning guide, its applications, and the ethical considerations surrounding its adoption is crucial for responsible and sustainable development.
It is also essential to address the ethical implications, such as bias, privacy, and accountability, to ensure its beneficial impact on society. By utilizing the potential of ML responsibly and transparently a future where intelligent systems work in harmony with humans, driving innovation and progress in a multitude of domains can be built.